In a groundbreaking study, Anthropic has unveiled some of the secrets behind how large language models (LLMs) function, addressing issues such as their tendency to fabricate information and struggle with math. While these AI models were designed, built, and trained by humans, their internal mechanisms remained largely a mystery—until now. Anthropic's novel approach, inspired by brain-scanning techniques, offers insights into the decision-making processes within LLMs, revealing why they often hallucinate answers and exactly how they approach problem-solving, like calculating math problems.
The research introduced a method called circuit tracing to examine the Claude 3.5 Haiku LLM. The approach allows scientists to identify which components of a model are active during different tasks, much like observing brain activity through a scanner. This new understanding sheds light on why LLMs perform poorly in tasks like basic arithmetic. In one instance, Claude added approximate numbers and combined them in an unconventional manner to arrive at an answer, which it then claimed to have computed using a more typical method.
Surprisingly, the study indicates that LLMs do not solely predict the next word in a sequence. Instead, they may plan certain elements first, such as determining the rhyming word in a poem before elaborating the rest of the verse. This finding challenges previous assumptions about AI model operations and opens new areas for both research and application.
However, the complexity of LLMs is far from fully understood. Despite this research, decoding even small sections of these models remains labor-intensive, often requiring hours of human effort. The study also explored how LLMs manage their 'hallucination' problem. It's identified that certain features within the model—like recognizing a 'known entity'—can inadvertently trigger the generation of fake information.
These discoveries set the stage for future advancements in understanding and improving AI technologies. Nevertheless, the research underscores the limitation that we still know only a fraction of the operations occurring inside these models. By continuing to probe their inner mechanics, researchers hope to develop more accurate and reliable AI solutions that could mitigate hallucinations and enhance cognitive functions, leading to better AI governance and performance.
In summary, while Anthropic's work marks a significant stride towards demystifying AI models, it also highlights the vast terra incognita that remains in computational neuroscience. This innovative study is a promising initial step towards more transparent machine learning systems.
AD
AD
AD
AD
Bias Analysis
Bias Score:
15/100
Neutral
Biased
This news has been analyzed from 6 different sources.
Bias Assessment: The article presents the scientific findings and implications in a neutral and factual manner, focusing on the research and its implications rather than opinions or sensationalism. While it applauds the novelty and importance of the research, which is largely based on evidence and expert analysis, it does not delve into subjective or political commentary, resulting in a low bias score.
Key Questions About This Article
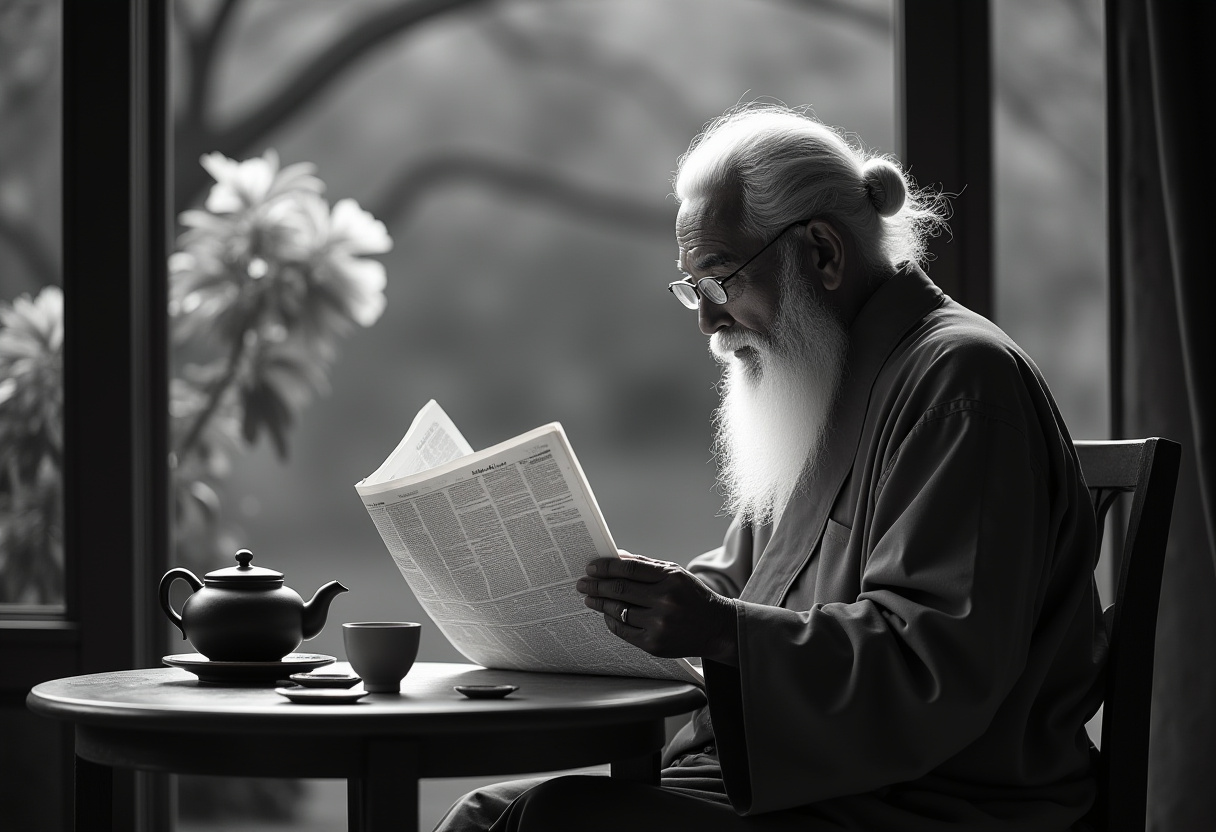